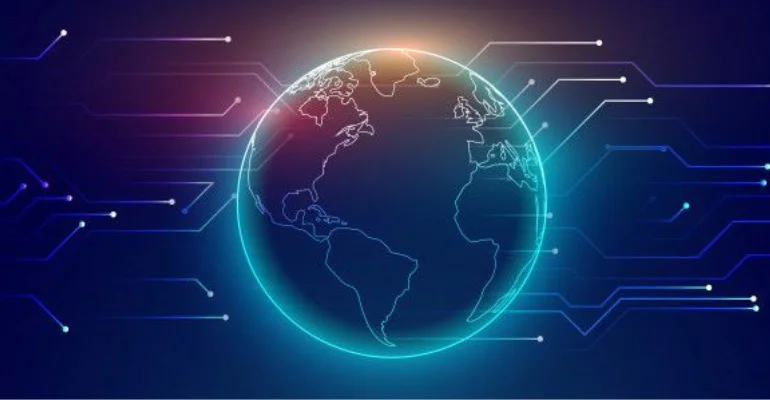
Data Privacy, Data Quality, Scalability
Addressing the challenges and considerations in leveraging AI for brand positioning involves a multifaceted approach, focusing on data privacy, data quality, scalability, and correct interpretation of data.
Data Privacy
Data privacy is a critical concern when collecting data for AI applications. AI technologies, such as large language models (LLMs) and generative AI projects, often ingest vast quantities of potentially sensitive data, including personally identifiable information (PII) and confidential company details. Compliance with data privacy regulations like the General Data Protection Regulation (GDPR) and the California Consumer Privacy Act (CCPA) is essential. Additionally, new AI-related directives, such as the EU’s AI Act and the US’s HTI-1 rule, impose further restrictions on data use for AI training and operation. To mitigate AI data privacy challenges, companies must adopt clear, comprehensive data privacy policies that can adapt to a shifting compliance landscape. Utilizing AI-powered data screening services can streamline compliance and reduce the impact on quality and efficiency .
Data Quality
Ensuring the quality and accuracy of data is crucial for AI systems to produce reliable results. The varying quality and accuracy of data from different sources necessitate robust AI systems capable of handling random data and distinguishing between reliable and less reliable sources. This involves implementing data quality management practices and leveraging AI technologies to automatically find and remove sensitive information from datasets, despite the challenges of false positives and the compute-intensive nature of such solutions .
Scalability
As the volume of incoming data increases, AI solutions must be scalable to maintain effectiveness. Scalability issues arise when dealing with large-scale unstructured datasets, requiring modern data governance solutions to ensure responsible and ethical use of AI technologies across different domains. Effective AI data governance involves data quality management, compliance with legal frameworks, and continuous monitoring, which help organizations navigate the complexities of AI deployment while adhering to ethical and legal standards .
Correct Interpretation
Extracting useful insights from multiple data sources can be complex, necessitating brands to develop expertise in interpreting results and turning data into actionable strategies. This involves understanding the nuances of AI outputs, including the potential for algorithmic bias and the importance of data accuracy in producing accurate outcomes. Companies must also consider data protection and control, ensuring that AI decisions are made fairly and with the knowledge, consent, and choice of the individuals involved .
In conclusion, while AI offers significant benefits for brand positioning, companies must address these challenges and considerations to ensure ethical, effective, and compliant use of AI technologies. This involves adopting comprehensive data privacy policies, implementing robust data quality management practices, ensuring scalability of AI solutions, and developing expertise in interpreting AI outputs. By navigating these challenges, companies can leverage AI to enhance their brand positioning strategies while maintaining compliance with legal and ethical standards.